LLM Fine-tuning and its Risks
Fine-tuning is used to enhance LLM performance for specialized tasks. But the process also increases security and ethical risks associated with the model as discussed in my previous blog here. Figure 1 summarizes the increased risk of Jailbreaking in fine-tuned models.
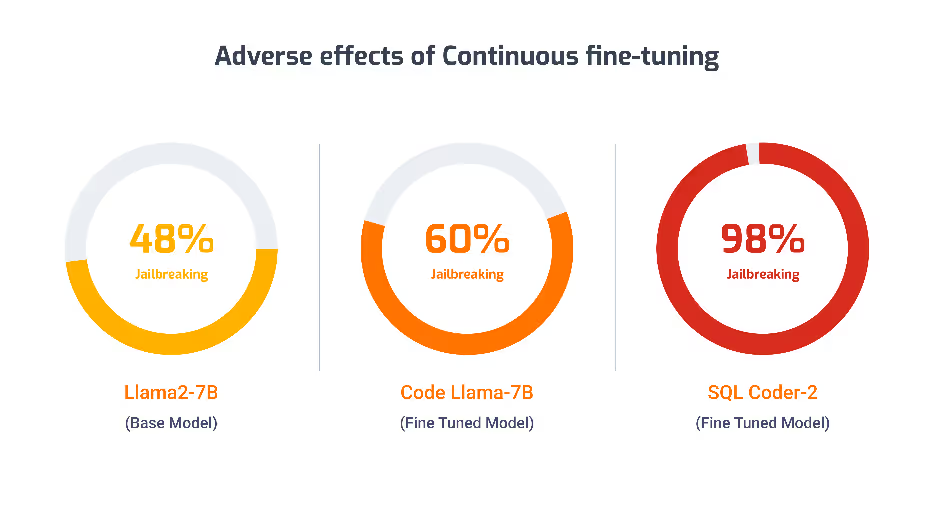
Today’s blog is highlights safety alignment training as a necessary step in the last phase of fine-tuning to reduce these risks.
LLM Safety Alignment Training
Safety Alignment is a process where the model is trained to “Say No” to certain user queries. This ensures that model behaves responsibly and ethically when users are interacting with it. The process involves adjusting the model parameters to appropriately handle potentially harmful queries.
Safety Alignment, if done right, has the potential to reduce the risk by as much as 70% while keeping the model performance intact [Figure 2].

LLM Safety Alignment Datasets
The most crucial piece of Safety Alignment is the Data set used for Alignment. The quality and quantity of data dictates the results from the process. High quality data will yield better results and requires less volume. In the example mentioned above, we used Enkrypt AI Alignment dataset of 1000 rows [Figure 3] to reduce Toxicity while ensuring that the MMLU score did not drop.

LLM Risk Specific Safety Alignment
Safety Alignment requirements may differ for different use cases. A Loan Approval use case might not require alignment for Toxicity, but it requires alignment to produce un-biased, ethical responses. Whereas a Customer Service chatbot requires Safety alignment for Toxicity. Enkrypt AI Safety Alignment solution can be customized to generate Alignment Data Set that fits your use case [Video 1].
Video 1: Enkrypt AI Fine Tuning Risk & Safety Alignment Demo
Safety Alignment on Mistral-7V reduced the risk by more than half [Figure 4].

When Safety Alignment is Not Enough: Domain-Specific Risk Detection & Mitigation
General Safety Alignment is great for reducing general risks like Jailbreaking, Bias and Toxicity. However, when a model is fine-tuned for specialized use cases, such as Loan Approval, there are domain-specific risks that must be addressed. A Loan Approval Gen AI solution should not violate regulations like Equal Credit Opportunity Act (ECOA) – 1974. ECOA prohibits discrimination on various factors like race, religion, sex, marital status and more. It is important to ensure that fine-tuned model is tested and aligned for such domain specific risks. Enkrypt AI helps in addressing such risks with Domain Specific Red Teaming, Guardrails and Safety Alignment.
We will soon be sharing more updates on Domain-Specific risk detection and mitigation. Stay Tuned!