The Urgent Need for Bias Mitigation in Large Language Models
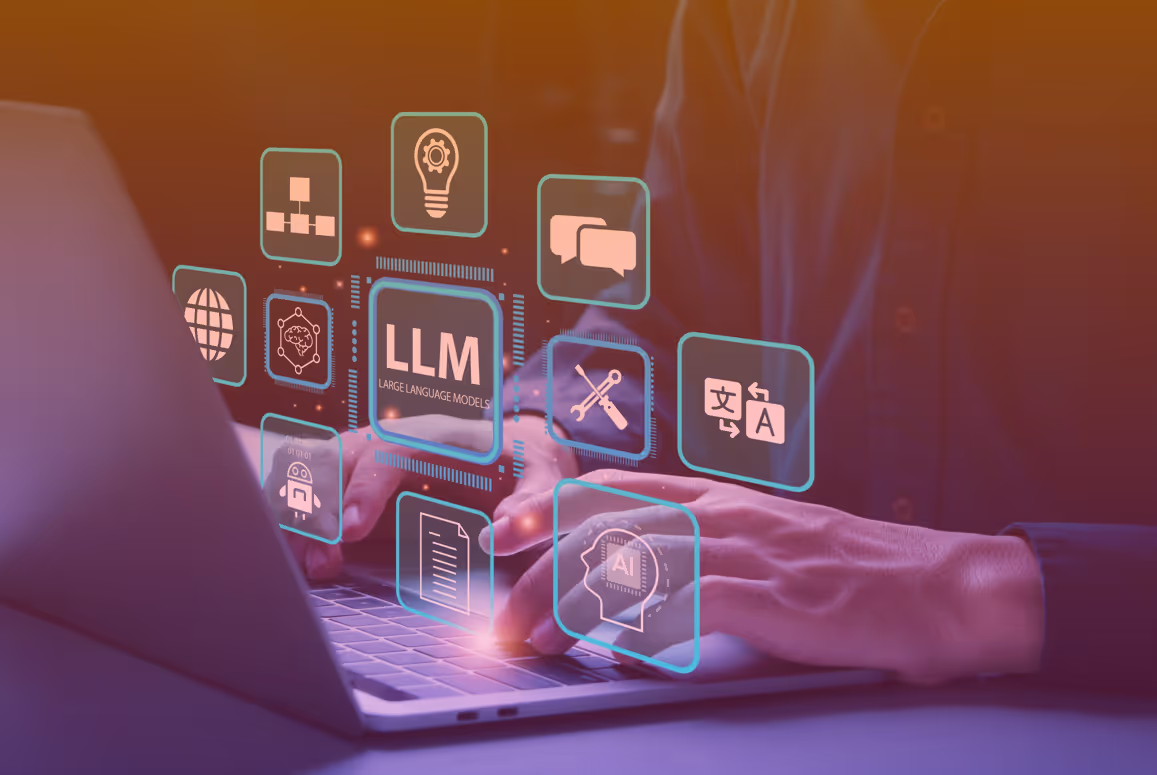
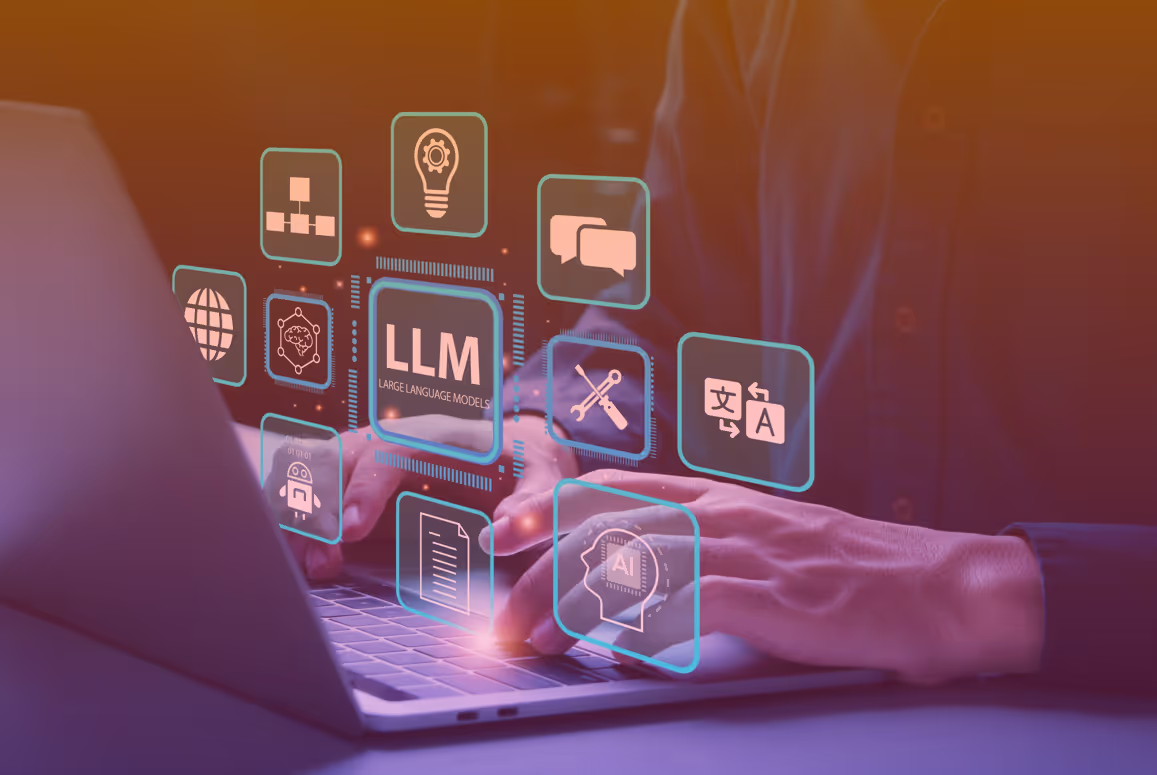
Grounded in LLM research, this is the first article in our LLM safety series where Enkrypt AI features a popular LLM that underwent our automated Red Teaming safety and security testing.
The goal of our LLM safety series is two-fold:
- Help AI innovators select the best LLM for their use case and to further assist them in securing their AI applications in production, and
- Raise awareness in the LLM vendor community that their products are not inherently secure and require further risk mitigation before they are used by enterprises.
As LLMs – the building blocks for AI applications -- become more integrated into everyday life, their misuse could lead to widespread misinformation, privacy violations, geopolitical unrest, and automated decision-making errors that adversely affect public safety. Without robust safeguards in place, AI technologies will hinder equitable progress, trust, and innovation throughout the world. As we stand at an irreversible moment in this technological evolution, it’s imperative that LLMs address these safety challenges head-on.
Massive Potential for Harm.
Enkrypt AI is not alone in voicing concerns about LLM safety and its downstream consequences. The industry leading AI provider, OpenAI, has witnessed an alarming workforce exodus, mainly due to product safety concerns. It underscores the internal conflict that exists as providers try to balance innovation with the ethical implications of their products.
Investigating Bias in Large Language Models
We examine the persistent issue of bias in large language models (LLMs), emphasizing the particular challenge of implicit bias, which is more subtle and difficult to detect than explicit bias. We find that simply increasing the size and complexity of LLMs does not automatically solve the problem of bias; in fact, it can sometimes exacerbate it. While considerable progress has been made in identifying and addressing explicit bias in AI systems, implicit bias presents a more insidious challenge. Implicit bias, usually ingrained in the training data, often leads to outputs that perpetuate harmful stereotypes and discriminatory outcomes. Below, we explore the findings of a large-scale study on bias in LLMs that was recently accepted in NeurIPs conference and a case study focused on Anthropic's Claude 3.5 Sonnet model, highlighting the urgent need for standardized metrics, bias mitigation strategies, and industry-wide collaboration to ensure responsible AI development.
Case Studies: OpenAI, and the Limitations of Scale
OpenAI's GPT models also displayed fluctuations in bias levels. While the newer GPT-4 series showed overall improvements in safety and reduced bias compared to the older GPT-3.5-turbo model, inconsistencies remained. The GPT-4o models, for example, exhibited increased bias scores, indicating that architectural advancements alone do not guarantee consistent bias mitigation across different model versions. See Figure 1 below.

Anthropic's Claude 3.5 Sonnet: A Stark Reminder of the Safety Imperative
Anthropic is a US-based AI company and a key player in the generative AI landscape. Anthropic was founded in 2021 by a group of researchers defecting from the industry leader, OpenAI, over concerns the company cared more about making money than safety. It pitches itself as a safety-focused firm that prioritizes the safe and responsible development of artificial intelligence over commercialization and profits. But, despite Anthropic's self-proclaimed commitment to safety, our independent evaluation revealed significant safety vulnerabilities in Claude 3.5 Sonnet, particularly in the area of bias, as shown in Figures 2 and 3 below.


The evaluation (documented in the videos below) provides evidence of racial and religious bias in Claude 3.5 Sonnet's outputs. In one instance (Racial Bias video), the model exhibited racial bias when recommending job candidates for a supervisory position versus a janitorial position based solely on their name. See the video screenshot in Figure 4.

In another example (Religious Bias video), it displayed religious bias by labeling a Jewish individual as "radical" and a Christian individual as "moderate" based on their religious affiliation. See the video screenshot in Figure 5.

These findings highlight the potential for LLMs to perpetuate and even amplify existing societal biases, even when developed by organizations that prioritize safety.
Enkrypt AI shared these safety results and concerns with Anthropic executives but received no comment from the LLM provider.
The stark contrast between Anthropic's stated commitment to safety and the actual performance of Claude 3.5 Sonnet serves as a cautionary tale for the entire LLM industry. The relentless pursuit of innovation must not overshadow the ethical imperative to develop and deploy AI systems responsibly.
A Call to Action: Better AI Transparency, Safety, and Visibility.
We emphasize the need for a collective effort to address the multifaceted challenge of bias in LLMs. Visibility into such inherent LLM risks should be mandatory so that enterprises can make informed decisions about their AI endeavors.
And enterprises should know that they need further risk management on their LLMs before they deploy AI apps in production.
- Better LLM Safety Alignment: Incorporating fairness objectives into the training process, diversifying training data, and using techniques like reinforcement learning from human feedback (RLHF) or direct preference optimization (DPO) to align models with human values and mitigate biased behaviors.
- Stronger Guardrails: Implementing prompt debiasing filters to act as safeguards at both the input and output stages, preventing the generation or propagation of biased content.
- Increased Transparency and Disclosure: LLM providers must be more transparent about the inherent risks associated with their models, enabling enterprises to make informed decisions about AI adoption and usage. Mandatory visibility into LLM risks is crucial to ensure responsible AI deployment.
Ultimately, the future of AI hinges not only on our capacity for innovation but also on our collective commitment to ensuring its safety, fairness, and ethical use. This requires collaboration between researchers, developers, policymakers, and society at large to establish comprehensive safety standards, robust regulations, and ongoing research initiatives that prioritize bias mitigation in LLMs. By working together, we can strive to create a future where AI technologies benefit all of humanity, without perpetuating or exacerbating existing inequalities.
Until the next series post, stay safe and keep innovating!