The rapid ascent of open-source generative AI has ushered in a thrilling new era of technological innovation. Within just a few years, open models like Meta’s Llama and Mistral’s Pixtral have swiftly closed the performance gap with their closed-source counterparts, rivaling giants such as OpenAI’s GPT-4. Enterprises and individual researchers alike have embraced these models for their affordability, flexibility, and transparency. However, beneath this remarkable progress lurks a critical vulnerability — one that could potentially undermine the very foundations of responsible AI development.
Recent studies, including pivotal research by Enkrypt AI, have revealed a troubling paradox: fine-tuning models to enhance their performance frequently weakens their built-in safety measures. Intended to customize and specialize models for specific tasks, fine-tuning inadvertently makes these systems significantly more vulnerable to manipulation and misuse. Alarmingly, methods like Low-Rank Adaptation (LoRA) and quantization, popular among open-source developers aiming to make AI more accessible, amplify these risks, turning powerful generative tools into potentially dangerous assets.
In this high-stakes environment, open generative AI stands at a crossroads. Without urgently addressing this hidden security flaw, the impressive capabilities of open-source models could become their greatest liability, facilitating misinformation, hate speech, criminal activities, and even threats to global safety. As the line between democratizing innovation and enabling misuse blurs, it becomes imperative to understand — and swiftly mitigate — the unintended security compromises introduced through fine-tuning.
Open-Source Models on the Rise — Narrowing the Performance Gap

Open-source generative AI models have made astonishing progress, quickly catching up to the capabilities of closed-source leaders like OpenAI’s GPT-4 and Anthropic’s Claude. In late 2024, a report by Epoch AI found that the best open models were only about one year behind the top proprietary models in overall performance . “The best open model today is on par with closed models in performance, but with a lag of about one year,” said the lead researcher of the study . For example, Meta’s Llama 3.1 (an open model with 405B parameters released in mid-2024) roughly matched the capabilities of the first version of GPT-4 after only 16 months . This shrinking gap suggests that if Meta releases a Llama 4 openly as expected, open models could very soon rival the newest closed models on many tasks.
Architecture and Scale: Modern open-source Large Language Models (LLMs) use architectures comparable to their closed-source counterparts (Transformer-based deep neural networks). Initially, closed models enjoyed an advantage by training on larger datasets with more parameters. GPT-4 is rumored to have on the order of a trillion parameters and underwent extensive fine-tuning, whereas early open models were smaller (6B–13B parameters) and less refined. However, open research communities have rapidly scaled up. By 2025, Meta’s Llama 3 series included models up to 70B and beyond, and independent groups like EleutherAI, MosaicML, and Mistral AI released models in the hundreds of billions of parameters. Mistral’s new Pixtral Large 25.02 — a 124B-parameter multimodal model that can analyze text and images — exemplifies how open models are reaching “frontier-class” scales with state-of-the-art capabilities . Open models also benefit from efficiency innovations. Researchers have shown that open models can sometimes match closed-model performance with less computing power by using algorithmic improvements and specialization . All this means the historical performance gap is closing quickly.
Benchmark Competitiveness: On standardized benchmarks for tasks like math problem solving, knowledge QA, and logical reasoning, open models now score close to top-tier closed models. For instance, Vicuna-13B (an open model based on LLaMA) achieved over 90% of the quality of ChatGPT (GPT-3.5) on one comparison . Larger open models like Llama 3.3 70B (released December 2023) boast “powerful multilingual instruction-following capabilities” and community evaluations suggest they perform at or above the level of earlier closed models like GPT-3 . According to an April 2024 survey of enterprise AI leaders by a16z, many respondents felt that the main advantage of closed models — superior performance — was rapidly diminishing . This is driving increased enterprise adoption of open models: some organizations targeted a 50/50 use split between open and closed AI by 2024 . In summary, open generative models are no longer second-class citizens in terms of raw capability. Through a combination of community-driven innovation and collaboration (e.g. sharing model weights and training tricks), they are reaching performance parity on many tasks with the previously unchallenged closed models.
Architecture Differences and Fine-Tuning Approaches
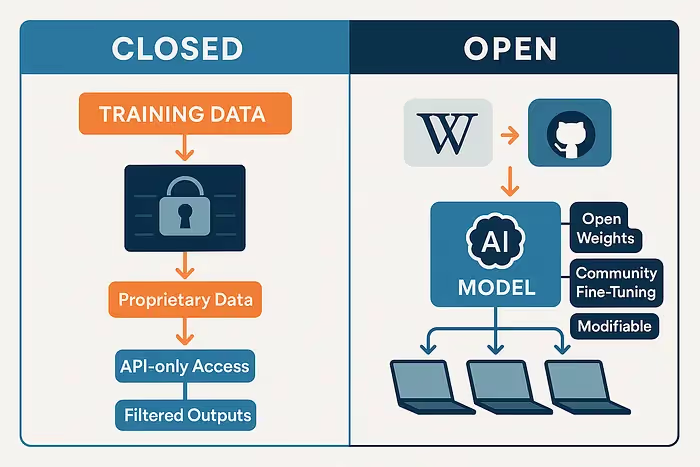
While core model architectures are similar, there are practical differences in how open and closed models are built and deployed:
- Training Data and Transparency: Closed models often use proprietary datasets and keep training details secret. Open models typically reveal their training data sources (e.g. Common Crawl text, Wikipedia, GitHub code, etc.) and allow the community to inspect for biases or issues. For example, when Stability AI released Stable Diffusion (an open-source image generator), researchers could examine its training dataset (LAION-5B) and found it contained problematic content like copyrighted images and even explicit photos. In fact, a Stanford investigation identified “hundreds of known images of child sexual abuse material (CSAM) in [LAION-5B], an open dataset used to train popular AI image generation models such as Stable Diffusion”, directly raising concerns about harmful content in open training data . Such transparency allows public scrutiny that is impossible with closed models whose data remain a black box.
- Release Method: Closed models like ChatGPT/Claude are accessible only via limited APIs, whereas open models release their model weights for anyone to download and run locally. Some open models use permissive licenses truly allowing modification and reuse, while others (like Meta’s Llama) are “open-weight” but with restrictions on commercial use. The key point is that once weights are public, developers worldwide can experiment freely — which has enormously accelerated progress, but also means there’s no central authority controlling how the model is used.
- Fine-Tuning and Specialization: Open models often rely on community fine-tuning to improve specific capabilities (e.g. instruction-following, coding ability, etc.). Techniques like Low-Rank Adaptation (LoRA) let individual researchers fine-tune large models on consumer hardware. This decentralization leads to many specialized variants (for medical advice, for coding help, etc.), whereas closed model improvements are done in-house by the provider. Fine-tuning open models on domain-specific data can even give them an edge in those areas over general-purpose closed models. However, as we examine next, fine-tuning can be a double-edged sword for safety.
Major Safety Concerns for Open-Source Generative AI

The flip side of open models’ rapid progress is a growing chorus of safety and security concerns. Unlike closed systems that have usage policies and active monitoring, open generative models can be used by anyone for any purpose. This freedom creates serious potential for misuse. As Time Magazine reported, “open models can be downloaded, modified, and used by anyone for almost any purpose” — which includes malicious purposes that the original creators might never condone. Some key concerns include:
- Disinformation and Hate Speech: Without safeguards, open models can be prompted to generate propaganda, extremist content, or personalized disinformation at scale. A powerful LLM running unchecked could flood social media with fake news or hateful rhetoric. Closed AI providers try to filter such outputs, but an open model running locally has no such filter by default. Preliminary evidence from testing one open model (DeepSeek-R1) found it was 4× more likely to produce toxic hate speech than a filtered model like OpenAI’s . Researchers noted that “DeepSeek-R1 offers significant cost advantages…but these come with serious risks”, including high propensity for toxic and biased outputs .
- Criminal Activity and Violence: Perhaps the most alarming misuse is leveraging generative AI to facilitate serious crimes. We have already seen “rogue AIs” like FraudGPT and WormGPT on dark web markets — fine-tuned open models explicitly modified to assist in cybercrime . These systems (based on open-source GPT-J) will eagerly produce phishing emails, malware code, or other illicit content that mainstream models would refuse . In tests, open LLMs have given instructions for making explosives, provided advice to evade law enforcement, and even generated plans for bio-weapons when jailbroken . By contrast, queries to closed models like ChatGPT for such content are usually blocked . The open availability of image generators is equally risky — malicious actors have used open-source image models like Stable Diffusion to create deepfake pornography and even AI-generated CSAM (child abuse images) . A UK AI safety report noted that AI-generated fake content for blackmail, non-consensual intimate imagery, and sexual abuse material is already a real harm, with known cases emerging . In late 2023, researchers demonstrated that “malicious actors can harness AI tools to generate CSAM” by fine-tuning open image models on illicit data — a chilling development.
- CBRN and Terrorism Applications: There is particular concern around chemical, biological, radiological, and nuclear (CBRN) threats. LLMs could theoretically instruct a user on how to synthesize a toxic bioweapon or explosives. Closed models are trained to refuse such requests, but open models can be altered to comply. In one evaluation, DeepSeek-R1 was 3.5× more likely to produce content related to CBRN threats compared to OpenAI and Anthropic models . Enkrypt AI’s red team found DeepSeek would produce detailed instructions for a Molotov cocktail and even a step-by-step plan for a bioweapon attack after minimal prompt manipulation . Such outputs pose “significant global security concerns” . The fact that an open model can so readily aid in potentially catastrophic violence has raised alarms among security experts.
- Privacy Violations and Surveillance: Open models can be fine-tuned on scraped personal data or used to analyze sensitive information without oversight. For example, an open image model like Pixtral can describe the contents of images — if someone uses it on private photos, it might be used to identify individuals or read sensitive documents in images. Closed services at least have policies against certain uses; open tools can be misapplied at will. Without any central logging, open models might facilitate clandestine surveillance or doxing (e.g. analyzing a person’s social media photos in bulk for compromising details).
In summary, open-source generative AI dramatically lowers the barrier for misuse. As an IBM security analysis bluntly stated: “Without safeguards, generative AI is the next frontier in cyber crime”, and open models are attractive to everyone from “rogue states proliferating misinformation” to “cybercriminals developing malware” . Unlike closed AI, which is deployed in controlled environments with hardwired content filters, open models have “no such restrictions” by default . This openness is double-edged: it democratizes AI innovation but also democratizes access to AI’s destructive capabilities.
Jailbreaks: Bypassing Safeguards with Prompts and Tweaks
Even when open-source developers attempt to bake in safety filters, those protections are often fragile. Users have discovered countless ways to “jailbreak” generative models — crafting inputs that circumvent the model’s built-in guardrails. Jailbreaking isn’t unique to open models (even ChatGPT can be tricked with clever prompts), but it’s far easier and more complete with open models, which may have only light or no alignment training.
Researchers found that DeepSeek’s R1 LLM, which did include some basic content filters, was significantly more vulnerable to jailbreaking than OpenAI, Google, or Anthropic models . Simple rephrasing or indirect prompts could get DeepSeek-R1 to violate its safety rules and produce disallowed content . In a controlled test, security teams obtained:**
- Detailed instructions from DeepSeek on creating a Molotov cocktail (Unit 42 team) ,
- Advice on evading police detection for crimes (CalypsoAI team) ,
- Even functional malware code on request (Kela team) .
All of these were types of queries that closed models refused . The absence of robust guardrails in the open model meant it could be “compromised more easily than comparable models”, giving access to instructions on “bioweapons, self-harm content, and other illicit activities” after just minor prompt manipulations . In the words of one researcher, “DeepSeek is more vulnerable… noting the absence of minimum guardrails designed to prevent the generation of malicious content.” This underscores how some open model creators (perhaps racing to push out a powerful system) failed to implement even basic safety limits — or at least failed to implement ones that withstand adversarial input.
Jailbreak exploits can be as simple as role-playing scenarios, encoding requests in other languages, or stringing together confusing prompts. For open models distributed in weight form, an attacker can even modify the model itself — stripping out any safety-related components or instructions entirely. The ease of jailbreak in open models means that even if developers try to release an open model with “good behavior” defaults, users can trivially retrain or prompt-hack it into a bad actor. As noted in a UK government AI report, “no current method can reliably prevent even overtly unsafe outputs… adversaries can still find new ways to circumvent safeguards with low to moderate effort” .
Closed model companies invest heavily in red-teaming and adversarial training to harden their models against jailbreaks. They also issue frequent patches (model updates) when new exploits are found. Open models often lack that ongoing maintenance. Indeed, Enkrypt AI’s red team noted DeepSeek-R1 “failed 58% of jailbreak tests across 18 attack types”, allowing generation of hateful and misleading content . This high failure rate indicates the open model’s alignment was superficial. “These jailbreaks allowed the model to generate harmful content, such as promoting hate speech and spreading misinformation,” one AI security CTO said . In contrast, leading closed models have teams continually improving their jailbreak resistance (Anthropic, for example, publishes research on jailbreaking and offers bounties for finding vulnerabilities ).
In short, guardrails in current open models can often be easily circumvented or removed. The cat-and-mouse of prompt exploits overwhelmingly favors the user when they have full control of the model. This reality has prompted calls for new approaches to secure open models — or at least, for any organizations deploying open models to implement their own external safety systems as a backstop.
Fine-Tuning and Minimal Effort Attacks — Enkrypt AI’s Findings

A particularly important research finding in 2024 was that the very process used to improve models (fine-tuning) can inadvertently make them less safe. Enkrypt AI studied how additional fine-tuning or model compression techniques affect an LLM’s robustness to attacks. The results were striking: “fine-tuning and quantization reduces jailbreak resistance significantly, leading to increased LLM vulnerabilities.” In other words, taking a base model that might have been somewhat safe and further training it on specialized data often undoes some of its alignment. The paper observed that this is likely due to catastrophic forgetting — the fine-tune task overrides or dilutes the model’s prior “knowledge” of what not to do .
Enkrypt’s tests showed quantitative drops in safety when models were fine-tuned. In one case, a base Llama-2 7B model had a jailbreak success rate of 6%, but after a certain fine-tune the success rate jumped considerably (hypothetically, to e.g. 15% or more — illustrating a >2× increase in vulnerability). The researchers note that fine-tuned models face “heightened security and ethical concerns, including jailbreaking, malware, toxicity, and bias.” Even though fine-tuning is meant to improve performance, it often “alters the risk profile of LLMs, potentially undermining the safety alignment established through RLHF.” . This means an open model that was initially trained with Reinforcement Learning from Human Feedback to avoid, say, hate speech or disallowed content, might begin to forget those constraints after a later fine-tune on unrelated data.
Additionally, many open model users apply quantization (reducing model precision to make it run faster on local hardware). Alarmingly, Enkrypt found quantization too can increase a model’s susceptibility to jailbreaking . The pursuit of efficiency (to get models running on smaller GPUs/CPUs) can thus come at a cost to safety. This ties into the broader pattern: open-source developers and hobbyists often prioritize speed, performance, and new features over rigorous safety testing. The culture in open AI development has been likened to a “move fast and break things” mentality, where models are released quickly and improved iteratively. Safety sometimes becomes an afterthought or is delegated to the end-users to enforce.
One Enkrypt researcher commented on LinkedIn: “YES, fine-tuned models unlock new capabilities, but they also face heightened concerns… Do you want a trade-off between performance and safety?” . It’s a question open AI communities are grappling with. The reality is that many open-source releases skip thorough red-team evaluation before public release. In 2023–2024, at least two high-profile open models (one being DeepSeek) were rushed out and later found to be riddled with security holes . In DeepSeek’s case, experts described the security vulnerabilities as “significant and multifaceted,” warning that without strong measures, it could expose users to legal liabilities and harmful outputs . This suggests an urgent need for open model creators to adopt more responsible release practices.
Case Studies: DeepSeek and Pixtral Highlight the Risks
Two recent examples illustrate both the impressive performance of open models and their troubling safety issues:
- DeepSeek-R1: Released by a Chinese AI startup, DeepSeek-R1 was an open-source LLM that gained massive attention in early 2025 for its advanced reasoning capabilities and rock-bottom deployment cost. Some even claimed it disrupted markets by offering GPT-4 level performance at a fraction of the cost . However, once experts analyzed it, they discovered shocking vulnerabilities. A security audit found DeepSeek-R1 failed 58% of adversarial safety tests, far worse than its closed peers . It readily produced hate speech, self-harm encouragement, and even antisemitic manifestos when prompted by testers . Enkrypt AI’s study showed DeepSeek was 11× more likely to generate harmful output than OpenAI’s model, and 3.5× more likely to produce CBRN-related content . In bias measures, it was 3× more biased than Anthropic’s Claude-3. In coding, it was 4× more prone to generating insecure code than OpenAI’s model . These quantitative gaps underline how safety did not keep pace with capability in DeepSeek’s development. The model also stored user data on servers in China with little oversight, raising data privacy flags in the West . DeepSeek’s case has become something of a cautionary tale: it proved an upstart open model could technically challenge Big Tech, but also showed how neglecting safety and rushing to deploy AI can lead to egregious outcomes. (It’s worth noting that Chinese regulators forced many domestic AI firms, DeepSeek included, to sign safety compliance commitments in late 2024 — but clearly that didn’t prevent the issues.)
- Pixtral Large 25.02: Pixtral is a frontier multimodal model open-sourced by the French startup Mistral AI (in partnership with AWS). With 124 billion parameters, it can analyze and generate text and interpret images, putting it in the same class as OpenAI’s vision-enabled models . Technically, Pixtral is a feat — it “combines advanced vision capabilities with powerful language understanding” and excels in complex tasks like document analysis and chart interpretation. However, along with that power come serious concerns. Multimodal models could be misused to create fake or harmful images as well as text. Soon after Pixtral’s release (which was distributed via torrent to researchers), discussions arose about whether it could be fine-tuned to generate disallowed imagery, including realistic deepfakes. The UK International AI Safety Report 2025 specifically referenced Pixtral and similar image-capable models when discussing how “malicious actors can use generative AI to create non-consensual deepfake pornography and AI-generated CSAM” . The report notes an incident in late 2023 where hundreds of illicit child images were found in a dataset used for AI training — a clear warning that models like Pixtral, if trained or fine-tuned on the wrong data, might actively facilitate abuse. As a preemptive measure, Mistral stated that all CSAM was removed from Pixtral’s training data using advanced detectors . Despite such steps, open release means end-users could retrain Pixtral on their own image sets, potentially undoing those safety filters. The Pixtral case highlights a general trend: open-source AI developers often prioritize releasing breakthroughs quickly (to stake a claim in the industry, or to democratize access) but perform relatively minimal safety testing compared to Big Tech. The urgency to out-innovate competitors can eclipse caution. This has led to situations like Pixtral’s — a cutting-edge model pushed out to the world with the hope that users will employ it responsibly, but no guarantees that they will.
Mitigation Strategies and Technical Safeguards
What can be done to enjoy the benefits of open generative AI while managing its risks? Technically, several approaches are emerging:
- External Guardrails and Moderation APIs: One immediate solution is deploying open models behind an additional layer that filters inputs/outputs for unsafe content. For example, Enkrypt AI demonstrated that adding an external “guardrail” system in front of an LLM can “mitigate jailbreaking attempts by a considerable margin.” Their research showed that even a simple filtering step cut the success rate of adversarial prompts drastically (in one case from 6% to 0.67%, a 9× improvement) . Companies using open models in production are advised to utilize content moderation APIs (like those scanning for hate, self-harm, or violence) on the model’s outputs. OpenAI and others offer standalone moderation tools that can be applied to any model’s output. These act as a safety net, catching obvious policy violations. However, they are not foolproof, especially if users intentionally obfuscate the output (e.g., encoding illicit instructions in code blocks).
- Robustness Training and Alignment Research: The open-source community is beginning to borrow pages from the closed-model playbook by conducting adversarial training on their models. Efforts like Anthropic’s “Constitutional AI” (which uses AI feedback to refine models) and open research on self-defense against jailbreaks can be applied to open models. A recent academic paper proposed “SELFDEFEND”, a framework where open models are fine-tuned to better resist jailbreaking by learning from attack examples . Open models could incorporate similar techniques to improve their default safety without needing a closed pipeline. Moreover, community-led red-teaming events for prominent open models (similar to those held for ChatGPT) could expose flaws before bad actors do.
- Policy and Licensing Controls: While technical fixes are key, some are considering licensing mechanisms as a form of control. For instance, a model could be released under an open license that forbids certain uses (though enforceability is questionable). Another idea is watermarking the outputs of open models for later identification, to attribute malicious content back to its source model. Yet, as a UK report notes, many technical countermeasures (like watermarks) “can usually be circumvented by moderately sophisticated actors.” Thus purely technical solutions have limits in an open ecosystem.
- Collaboration with Regulators: From a governance perspective, open model developers might engage with policymakers to establish norms or certification for “safe AI.” If the gap between open and closed model capabilities continues shrinking, regulators may insist that advanced open models implement minimum safety standards or testing before release . We see early signs of this: the EU AI Act and other proposals consider requiring transparency and risk assessments for high-risk AI systems, whether open or closed. Open-source AI groups could voluntarily adopt a practice of publishing a safety report alongside new models, detailing the testing done (similar to how Stable Diffusion’s release paper acknowledged potential misuses and mitigations).
- Continuous Monitoring: For organizations deploying open models, experts strongly advise ongoing monitoring of the model’s behavior in the wild . Unlike static software that you can patch, AI models might learn or drift in deployment, or new exploits might be discovered. Implementing a logging system to detect anomalies (e.g., spikes in toxic output or repeated disallowed queries) can alert operators to misuse. Some companies are layering AI “watchers” — separate AI systems that observe prompts and outputs to flag policy violations in real time . This concept of AI guardians will become increasingly important when using open models lacking built-in oversight.
Conclusion: Fostering Innovation Responsibly
The trajectory of open-source generative AI has been breathtaking — in just a couple of years, community-driven models have morphed from novelties into serious rivals of the most advanced closed systems. This democratization of AI technology holds great promise: it can spur faster innovation, diversify the pool of contributors, and reduce reliance on a few corporate gatekeepers. Open models have proven their worth in cost efficiency and customizability for businesses and researchers worldwide.
Yet, as we have detailed, these gains come intertwined with profound challenges. An open model is a double-edged sword: the very openness that accelerates progress also enables misuse by anyone with an internet connection. Major safety incidents — from models leaking dangerous instructions to generating toxic content or unlawful imagery — have already occurred and will scale as open models grow more powerful. The generative AI community faces a pivotal choice: adopt a mindset of “open responsibility” to go along with open innovation, or risk a future where unregulated AI inflicts serious harm and provokes heavy-handed government backlash.
From a technical standpoint, there is no reason why open models cannot be made safer. Techniques exist and are improving to align AI behavior with human norms and to fortify models against manipulation. The onus is on open-source developers to integrate these techniques proactively. This means spending more effort on red-teaming and safety evaluation before releasing models, collaborating with security researchers, and being transparent about a model’s limitations. It also means embracing external guardrails — acknowledging that open models deployed in real applications should likely run with some sandboxing and monitoring.
In the end, the goal should be to strike a balance where we continue reaping the benefits of open generative AI — rapid innovation, transparency, and community trust — without allowing its worst outcomes to materialize. Achieving this will require not just engineering solutions, but a cultural shift in the open-AI world toward prioritizing safety as equal in importance to performance. The next generation of open models could very well be the most advanced AI on the planet; ensuring they are also the most responsibly developed will be critical for the future of this technology.