The Top 3 Trends in LLM Security Gathered from 10 AI Events in 2 Months


As CEO and Co-Founder of Enkrypt AI, I’m on an extensive travel schedule to network, promote our solution, and learn what motivates executives on AI security. In the last two months, I’ve attended over 10 events – including partner-sponsored sessions, regional summits, and large vendor-sponsored conferences like Gartner. And I’ve learned that everyone has the same goal: to accelerate AI adoption in a secure manner while retaining competitive advantage and minimize brand damage.
So, after listening to countless hours of presentations and hearing audience feedback, here are the 3 major trends that keep emerging when it comes to LLM security.
1 - Security versus Risk
The choice between security and risk is an easy decision – especially if you are a C-level executive. With the ever-growing number of threats emerging daily, it’s a foregone conclusion that you’ll be hacked. It’s just a matter of time. What matters more is the risk impact from those breaches and the degree of difficulty it will be to recover – both financially and legally.
To best manage risk, you should choose a solution that dynamically detects and removes LLM vulnerabilities. Your risk posture improves even more if you can show cost savings for such actions in real-time.
Instead of plugging numbers into complex, theoretical ROI models to justify spend on various cyber security tools, you should be able to see your specific risk scores that translate into actual costs savings. A dashboard view of such a solution can be seen in Figure 1.
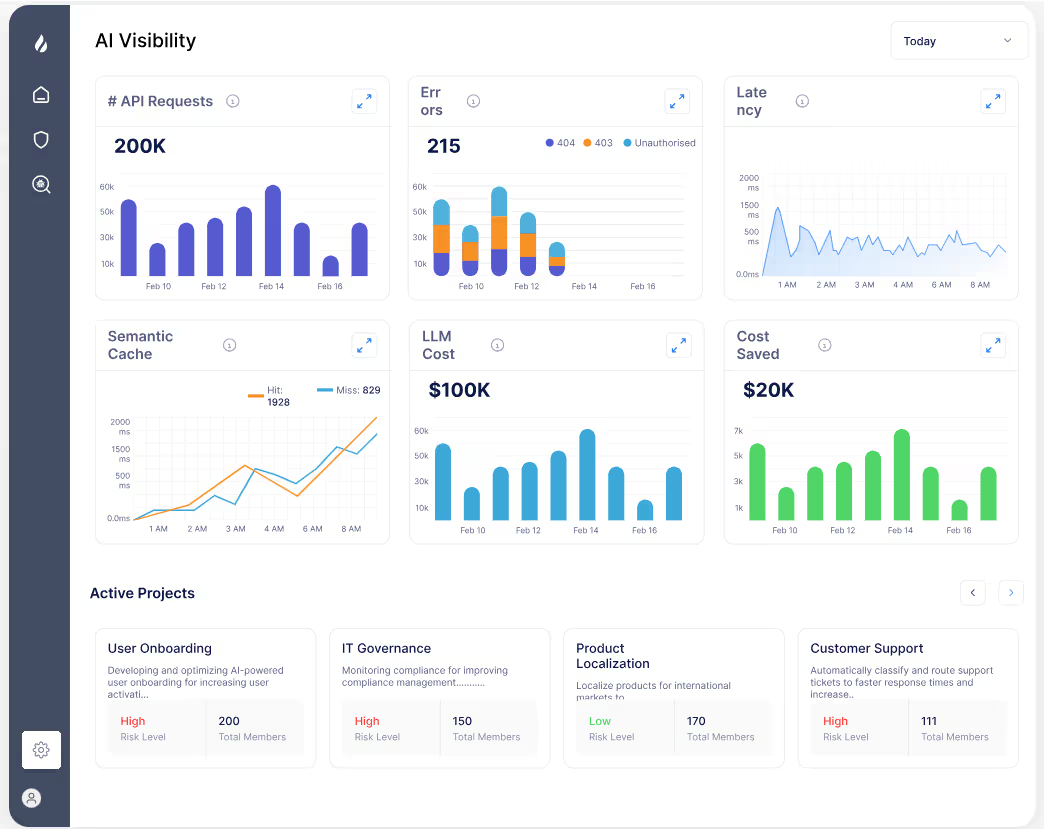
2 – Risk Tolerance Varies by Vertical
Another trend I’ve seen is the huge differences that exist in risk tolerance depending on the industry. For example, one of our Life Sciences customers is laser focused on their custom-built AI chatbot. They get daily reports from us on the bias, toxicity, malware, and jailbreaking risk scores from this use case alone to ensure an optimal patient experience.
The same goes for our insurance client. They are maniacal about the safe and compliant use of AI for processing sensitive claims data. Their custom LLM is more prone to bias than industry averages, so we do further testing and alignment to ensure information is accurate.
How can you ensure your risk is constantly reducing the more your AI is used? Find a solution that doesn’t just test against the usual threat suspects, but one that can surface vulnerabilities from additional prompting against your internal and external data sets. So over time, your AI applications become safer, improving your risk profile and corporate social responsibility. Refer to Figure 2 for an example.
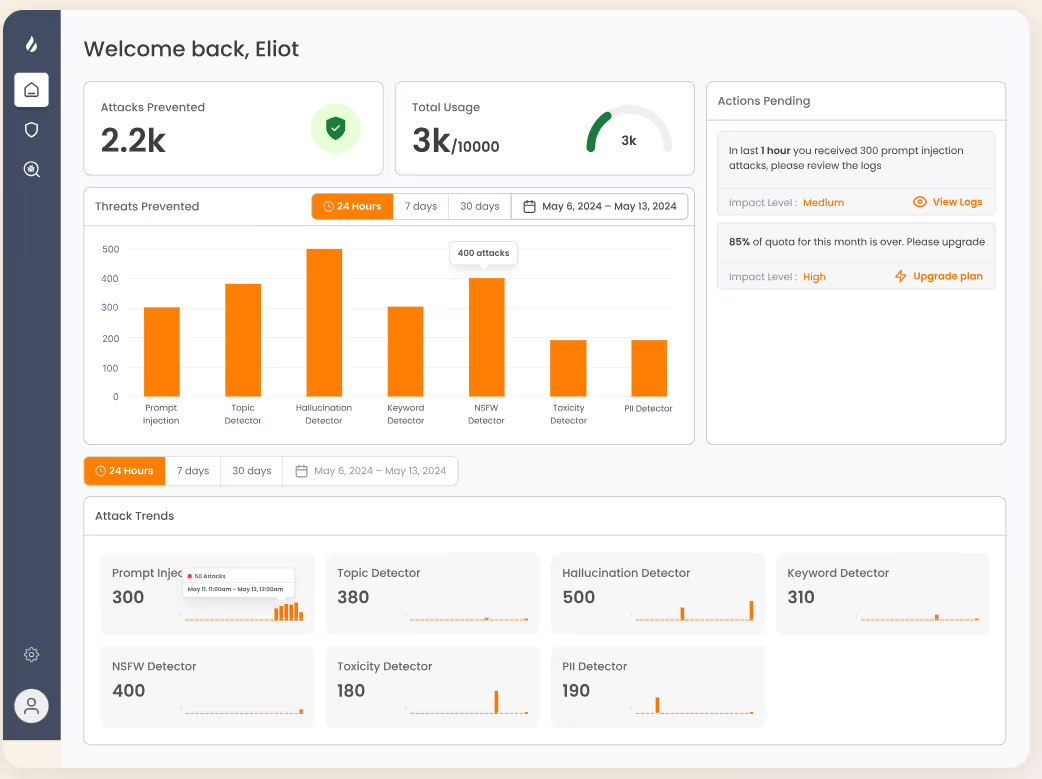
3 - Minimum Requirement: Red Teaming
AI experts agree: if you had to choose just one thing to improve your AI security posture, then do Red Teaming testing (i.e. AI threat detection). It’s the biggest trend I’ve been hearing for over the past year, with the audience voices becoming louder in the last few months.
There are obvious differences in testing services when it comes to Red Teaming. One big thing we suggest is conducting both dynamic and static testing.
Dynamic testing involves injecting a variety of real-time, iterative prompts that become increasingly sharper based on the LLM use case. Such methods detect significantly more vulnerabilities than static testing alone. Refer to Figure 3 below.

About Enkrypt AI and Its Automated Controls
Keeping in mind these 3 LLM security trends, know that we can help organizations with these goals and far more. Contact us today to get a demo of our comprehensive platform. Once you deploy Enkrypt AI, the product automatically scans and protects your LLMs – no manual work is required. This “set it and forget it” approach allows you to focus on innovation rather than worrying about security. You’ll also realize cost savings of $1-2M / year with every vulnerability detected and removed.